Utilization of Artificial Intelligence in Breast Pathology
An Overview
DOI:
https://doi.org/10.21141/PJP2024.04Keywords:
AI algorithm, anatomicpathology, artificial intelligence, breast cancer, digitized slides, whole slide imagesAbstract
In the last decade, artificial intelligence (AI) has been increasingly used in various fields of medicine. Recently, the advent of whole slide images (WSI) or digitized slides has paved the way for AI-based anatomic pathology. This paper set out to review the potential integration of AI algorithms in the workflow, and the utilization of AI in the practice of breast pathology.
Downloads
References
World Health Organization. Breast cancer. https://www.who.int/news-room/fact-sheets/detail/breast-cancer. Accessed on March 9, 2024.
Wu TY, Lee J. Promoting breast cancer awareness and screening practices for early detection in low-resource settings. Eur J Breast Health. 2018; 15(1):18-25. https://pubmed.ncbi.nlm.nih.gov/30816360. https://www.ncbi.nlm.nih.gov/pmc/articles/PMC6385717. https://doi.org/10.5152/ejbh.2018.4305.
Mayo Clinic. Breast cancer. https://www.mayoclinic.org/diseases-conditions/breast-cancer/diagnosis-treatment/drc-20352475. Accessed on March 9, 2024.
Meroueh C, Chen ZE. Artificial intelligence in anatomical pathology: building a strong foundation for precision medicine. Hum Pathol. 2023; 132:31-8. https://pubmed.ncbi.nlm.nih.gov/35870567. https://doi.org/10.1016/j.humpath.2022.07.008.
Sandbank J, Bataillon G, Nudelman A, et al. Validation and real-world clinical application of an artificial intelligence algorithm for breast cancer detection in biopsies. NPJ Breast Cancer. 2022; 8(1):129. https://pubmed.ncbi.nlm.nih.gov/36473870. https://www.ncbi.nlm.nih.gov/pmc/articles/PMC9723672. https://doi.org/10.1038/s41523-022-00496-w.
Roth J, Taatjes DJ. Histochemistry and cell biology-a glance into the past and a look ahead. Histochem Cell Biol. 2023; 159(6):465-75. https://pubmed.ncbi.nlm.nih.gov/37195292. https://www.ncbi.nlm.nih.gov/pmc/articles/PMC10247834. https://doi.org/10.1007/s00418-023-02195-4.
Pavlisko EN, Howell DN. The continued vital role of electron microscopy in the diagnosis of renal disease/dysfunction. Ultrastruct Pathol. 2013; 37(1):1-8. https://pubmed.ncbi.nlm.nih.gov/23383611. https://doi.org/10.3109/01913123.2012.670025.
Vallat JM, Richard L, Sindou P, Magy L. Electron microscopy as a tool for the diagnosis of neuropathies. Ann Pathol. 2008; 28(6):486-94. https://pubmed.ncbi.nlm.nih.gov/19084717. https://doi.org/10.1016/j.annpat.2008.04.006.
Malone ER, Oliva M, Sabatini PJB, Stockley TL, Siu LL. Molecular profiling for precision cancer therapies. Genome Med. 2020; 12(1):8. https://pubmed.ncbi.nlm.nih.gov/31937368. https://www.ncbi.nlm.nih.gov/pmc/articles/PMC6961404. https://doi.org/10.1186/s13073-019-0703-1.
Bera K, Schalper KA, Rimm DL, Velcheti V, Madabhushi A. Artificial intelligence in digital pathology - new tools for diagnosis and precision oncology. Nat Rev Clin Oncol. 2019; 16(11):703-15. https://pubmed.ncbi.nlm.nih.gov/31399699. https://www.ncbi.nlm.nih.gov/pmc/articles/PMC6880861. https://doi.org/10.1038/s41571-019-0252-y.
Yao K, Singh A, Sridhar K, Blau JL, Ohgami RS. Artificial intelligence in pathology: a simple and practical guide. Adv Anat Pathol. 2020; 27(6):385-93. https://pubmed.ncbi.nlm.nih.gov/32773432. https://doi.org/10.1097/PAP.0000000000000277.
Mi W, Li J, Guo Y, Ren X, Liang Z, Zhang T, Zou H. Deep learning-based multi-class classification of breast digital pathology images. Cancer Manag Res. 2021;10;13:4605-17. https://pubmed.ncbi.nlm.nih.gov/34140807. https://www.ncbi.nlm.nih.gov/pmc/articles/PMC8203273. https://doi.org/10.2147/CMAR.S312608.
Lu Z, Zhan X, Wu Y, et al. BrcaSeg: A deep learning approach for tissue quantification and genomic correlations of histopathological images. Genomics Proteomics Bioinformatics. 2021;19(6):1032-42. https://pubmed.ncbi.nlm.nih.gov/34280546. https://www.ncbi.nlm.nih.gov/pmc/articles/PMC9403022. https://doi.org/10.1016/j.gpb.2020.06.026.
Im S, Hyeon J, Rha E, et al. Classification of diffuse glioma subtype from clinical-grade pathological images using deep transfer learning. Sensors (Basel). 2021; 21(10):3500. https://pubmed.ncbi.nlm.nih.gov/34067934. https://www.ncbi.nlm.nih.gov/pmc/articles/PMC8156672. https://doi.org/10.3390/s21103500.
Hu Y, Su F, Dong K. et al. Deep learning system for lymph node quantification and metastatic cancer identification from whole-slide pathology images. Gastric Cancer 2021;24(4):868–77. https://pubmed.ncbi.nlm.nih.gov/33484355. https://doi.org/10.1007/s10120-021-01158-9.
Cheng S, Liu S, Yu J, et al. Robust whole slide image analysis for cervical cancer screening using deep learning. Nat Commun. 2021;12(1):5639. https://pubmed.ncbi.nlm.nih.gov/34561435. https://www.ncbi.nlm.nih.gov/pmc/articles/PMC8463673. https://doi.org/10.1038/s41467-021-25296-x.
Shin SJ, You SC, Jeon H, et al. Style transfer strategy for developing a generalizable deep learning application in digital pathology. Comput Methods Programs Biomed. 2021;198:105815. https://pubmed.ncbi.nlm.nih.gov/33160111. https://doi.org/10.1016/j.cmpb.2020.105815.
Zhou C, Jin Y, Chen Y, et al. Histopathology classification and localization of colorectal cancer using global labels by weakly supervised deep learning. Comput Med Imaging Graph. 2021;88:101861. https://pubmed.ncbi.nlm.nih.gov/33497891. https://doi.org/10.1016/j.compmedimag.2021.101861.
Salvi M, Bosco M, Molinaro L, et al. A hybrid deep learning approach for gland segmentation in prostate histopathological images. Artif Intell Med. 2021; 115:102076. https://pubmed.ncbi.nlm.nih.gov/34001325. https://doi.org/10.1016/j.artmed.2021.102076.
Kers J, Bülow RD, Klinkhammer BM, et al. Deep learning-based classification of kidney transplant pathology: A retrospective, multicentre, proof-of-concept study. Lancet Digit Health. 2022; 4(1): e18-26. https://pubmed.ncbi.nlm.nih.gov/34794930. https://doi.org/10.1016/S2589-7500(21)00211-9.
Shim WS, Yim K, Kim TJ, et al. DeepRePath: Identifying the prognostic features of early-stage lung adenocarcinoma using multi-scale pathology images and deep convolutional neural networks. Cancers (Basel). 2021;13(13):3308. https://pubmed.ncbi.nlm.nih.gov/34282757. https://www.ncbi.nlm.nih.gov/pmc/articles/PMC8268823. https://doi.org/10.3390/cancers13133308.
Pantanowitz L, Sinard JH, Henricks WH, et al; College of American Pathologists Pathology and Laboratory Quality Center. Validating whole slide imaging for diagnostic purposes in pathology: guideline from the College of American Pathologists Pathology and Laboratory Quality Center. Arch Pathol Lab Med. 2013; 137(12):1710-22. Phttps://pubmed.ncbi.nlm.nih.gov/23634907. https://www.ncbi.nlm.nih.gov/pmc/articles/PMC7240346. https://doi.org/10.5858/arpa.2013-0093-CP.
Kim I, Kang K, Song Y, Kim TJ. Application of artificial intelligence in pathology: trends and challenges. Diagnostics (Basel). 2022;12(11):2794. https://doi.org/10.3390/diagnostics12112794.
Retamero JA, Aneiros-Fernandez J, Del Moral RG. Complete digital pathology for routine histopathology diagnosis in a multicenter hospital network. Arch Pathol Lab Med. 2020; 144(2):221-8. https://pubmed.ncbi.nlm.nih.gov/31295015. https://doi.org/10.5858/arpa.2018-0541-OA.
Williams B, Hanby A, Millican-Slater R, et a. Digital pathology for primary diagnosis of screen-detected breast lesions - experimental data, validation and experience from four centres. Histopathology. 2020; 76(7):968-975. https://pubmed.ncbi.nlm.nih.gov/31994224. https://doi.org/10.1111/his.14079.
Campanella G, Hanna MG, Geneslaw L, et al. Clinical-grade computational pathology using weakly supervised deep learning on whole slide images. Nat Med. 2019;25(8):1301-9. https://pubmed.ncbi.nlm.nih.gov/31308507. https://www.ncbi.nlm.nih.gov/pmc/articles/PMC7418463. https://doi.org/10.1038/s41591-019-0508-1.
Liu Y, Kohlberger T, Norouzi M, et al. Artificial intelligence-based breast cancer nodal metastasis detection: insights into the black box for pathologists. Arch Pathol Lab Med. 2019 ;143(7):859-68. https://pubmed.ncbi.nlm.nih.gov/30295070. https://doi.org/10.5858/arpa.2018-0147-OA.
Serag A, Ion-Margineanu A, Qureshi H, et al. Translational AI and deep learning in diagnostic pathology. Front Med (Lausanne). 2019; 6:185. https://pubmed.ncbi.nlm.nih.gov/31632973. https://www.ncbi.nlm.nih.gov/pmc/articles/PMC6779702. https://doi.org/10.3389/fmed.2019.00185.
Pantanowitz L, Quiroga-Garza GM, Bien L, et al. An artificial intelligence algorithm for prostate cancer diagnosis in whole slide images of core needle biopsies: a blinded clinical validation and deployment study. Lancet Digit Health. 2020; 2(8): e407-16. https://pubmed.ncbi.nlm.nih.gov/33328045. https://doi.org/10.1016/S2589-7500(20)30159-X.
da Silva LM, Pereira EM, Salles PG, et al. Independent real-world application of a clinical-grade automated prostate cancer detection system. J Pathol. 2021; 254(2):147-58. https://pubmed.ncbi.nlm.nih.gov/33904171. https://www.ncbi.nlm.nih.gov/pmc/articles/PMC8252036. https://doi.org/10.1002/path.5662.
Chen N. Leadership quote of the week: the one most adaptable to change is the one that survives https://laidlawscholars.network/posts/the-one-most-adaptable-to-change-is-the-one-that-survives. Accessed on March 7, 2024.
Schnitt S. AI in breast pathology: how can artificial intelligence improve breast cancer diagnosis [Video]. YouTube. https://www.youtube.com/watch?v=OJwq31VRsdU. February 20, 2023. Accessed on March 7, 2024.
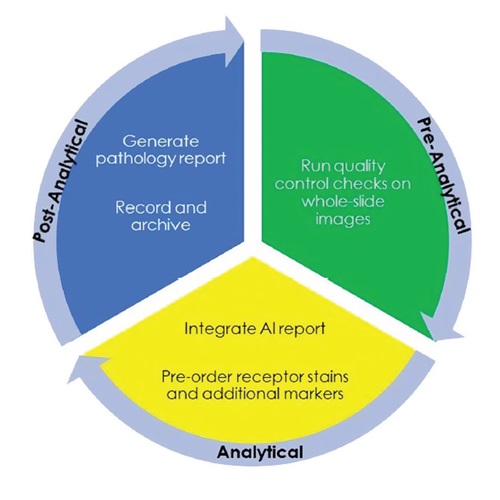
Downloads
Published
How to Cite
Issue
Section
License
Copyright (c) 2024 PJP

This work is licensed under a Creative Commons Attribution-NonCommercial-ShareAlike 4.0 International License.

The Philippine Journal of Pathology is licensed under a Creative Commons Attribution-NonCommercial-ShareAlike 4.0 International License. Based on works made open access at http://philippinejournalofpathology.org